Decision Trees
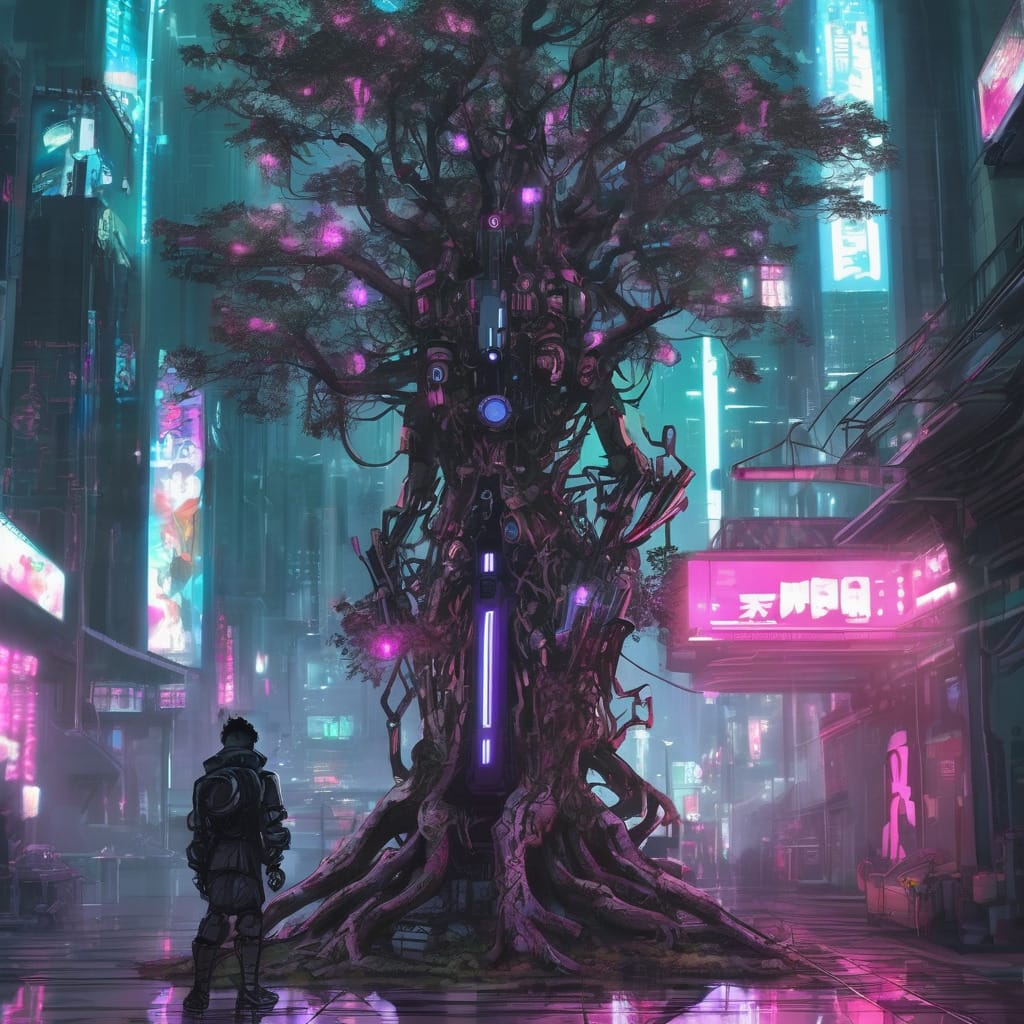
Decision Trees are a type of machine learning algorithm that uses a tree-like structure to classify data or make predictions. They are a popular algorithm for classification and regression tasks, and are known for their simplicity and interpretability.
What is a Decision Tree?
A Decision Tree is a tree-like structure that splits the data into subsets based on the input features. Each internal node in the tree represents a feature or attribute, and each leaf node represents a class label or a predicted value.
How Does a Decision Tree Work?
A Decision Tree works by recursively partitioning the data into smaller subsets based on the input features. The algorithm starts at the root node, and at each internal node, it tests a condition on the input feature. Based on the outcome of the test, the algorithm moves to the left or right child node, and the process is repeated until a leaf node is reached.
Key Components of a Decision Tree
- Root Node: The root node is the top-most node in the tree, and represents the input features.
- Internal Nodes: The internal nodes represent the feature or attribute that is being tested.
- Leaf Nodes: The leaf nodes represent the class label or the predicted value.
- Edges: The edges represent the relationships between the nodes in the tree.
Types of Decision Trees
- Classification Trees: These trees are used for classification tasks, and the leaf nodes represent class labels.
- Regression Trees: These trees are used for regression tasks, and the leaf nodes represent the predicted value.
- Ensemble Methods: These methods combine multiple Decision Trees to improve the accuracy and robustness of the model.
Applications of Decision Trees
- Classification: Decision Trees are widely used for classification tasks, such as spam filtering and credit risk assessment.
- Regression: Decision Trees are used for regression tasks, such as predicting continuous outcomes like house prices.
- Feature Selection: Decision Trees can be used for feature selection, by identifying the most important features that contribute to the prediction.
Advantages of Decision Trees
- Easy to Interpret: Decision Trees are a simple and intuitive algorithm that is easy to interpret.
- Fast to Train: Decision Trees are a fast algorithm that can be trained quickly.
- Handles Categorical Features: Decision Trees can handle categorical features, which is a common type of data in many domains.
Disadvantages of Decision Trees
- Overfitting: Decision Trees can suffer from overfitting, especially when the tree is too deep or too complex.
- Not Suitable for Non-Linear Relationships: Decision Trees are not suitable for modeling non-linear relationships.
- Not Suitable for High-Dimensional Data: Decision Trees can be computationally expensive for high-dimensional data.
I hope this overview helps you understand Decision Trees better!