Gated Recurrent Unit (GRU) Networks
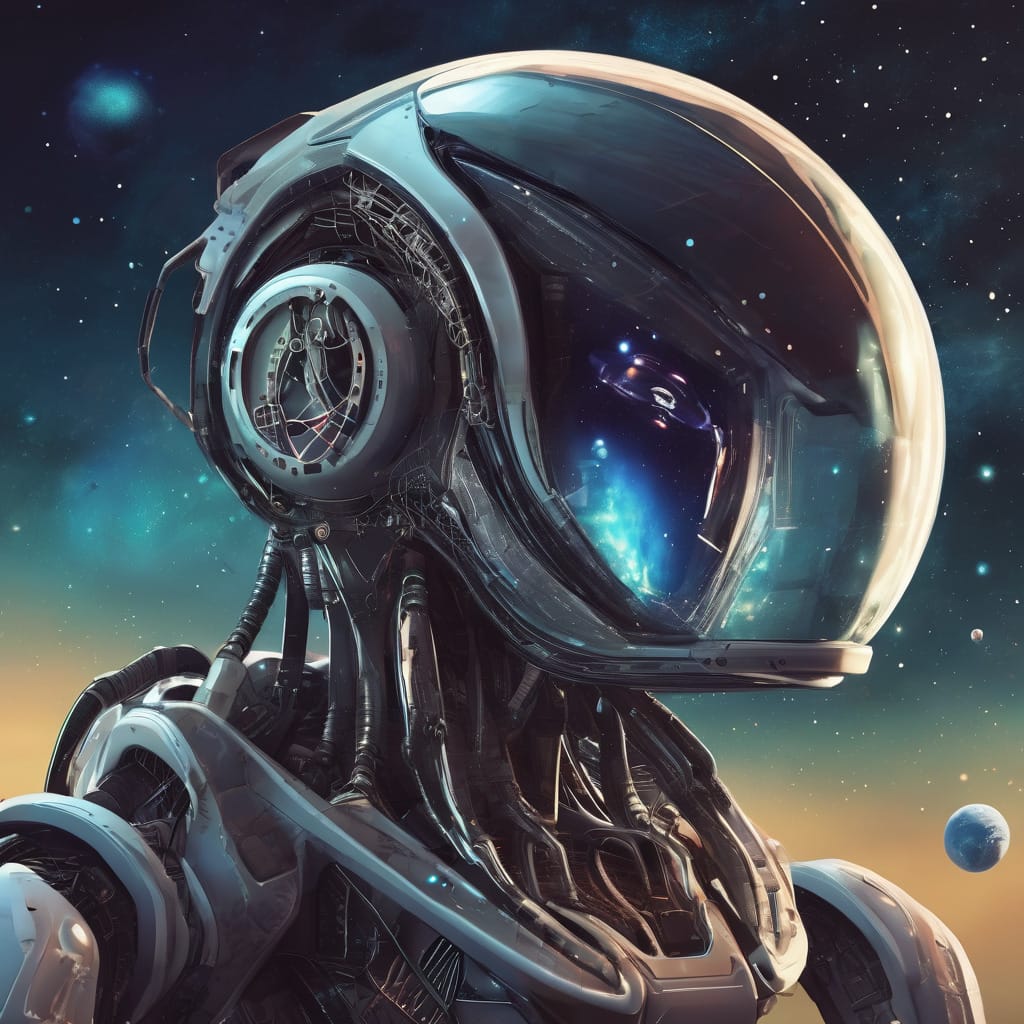
Gated Recurrent Unit (GRU) Networks are a type of Recurrent Neural Network (RNN) that is designed to handle sequential data, such as speech, text, or time series data. GRU Networks are characterized by their ability to handle long-term dependencies in data using a simpler architecture than LSTM Networks.
Key Components of GRU Networks
- Input Gate: The input gate controls the flow of information into the GRU Network.
- Reset Gate: The reset gate controls the flow of information out of the GRU Network.
- Hidden State: The hidden state stores information from previous time steps.
- Activation Functions: GRU Networks use activation functions such as sigmoid or tanh to introduce non-linearity into the network.
How GRU Networks Work
- Sequential Data: GRU Networks process sequential data one time step at a time.
- Hidden State: The hidden state stores information from previous time steps.
- Input Gate: The input gate controls the flow of information into the GRU Network.
- Reset Gate: The reset gate controls the flow of information out of the GRU Network.
Advantages of GRU Networks
- Ability to Handle Long-Term Dependencies: GRU Networks can handle long-term dependencies in data using a simpler architecture than LSTM Networks.
- Faster Training: GRU Networks train faster than LSTM Networks.
- Good Performance on Simple Tasks: GRU Networks perform well on simple tasks such as speech recognition and text processing.
Disadvantages of GRU Networks
- Difficulty in Handling Complex Patterns: GRU Networks struggle to handle complex patterns in data.
- Difficulty in Handling Large Datasets: GRU Networks can struggle to handle large datasets.
Applications of GRU Networks
- Speech Recognition: GRU Networks are used for speech recognition tasks, such as speech-to-text systems.
- Text Processing: GRU Networks are used for text processing tasks, such as language modeling and text classification.
- Time Series Forecasting: GRU Networks are used for time series forecasting tasks, such as predicting stock prices or weather patterns.
Future of GRU Networks
- Increased Use in Real-World Applications: GRU Networks are expected to be used increasingly in real-world applications, such as speech recognition and text processing.
- Improvements in Training Algorithms: Researchers are working on improving training algorithms for GRU Networks, such as using techniques like attention and memory-augmented networks.
- Increased Use in Multimodal Applications: GRU Networks are expected to be used increasingly in multimodal applications, such as speech and image processing.
Comparison with Other RNN Architectures
- Simple RNN: GRU Networks are more powerful than Simple RNNs, but require more computation.
- LSTM: GRU Networks are less powerful than LSTMs, but require less computation.
- Bi-Directional RNN: GRU Networks can be used in bi-directional RNNs, but require more computation.
I hope this helps! Let me know if you have any questions or need further clarification.