Large Language Models (LLMs)
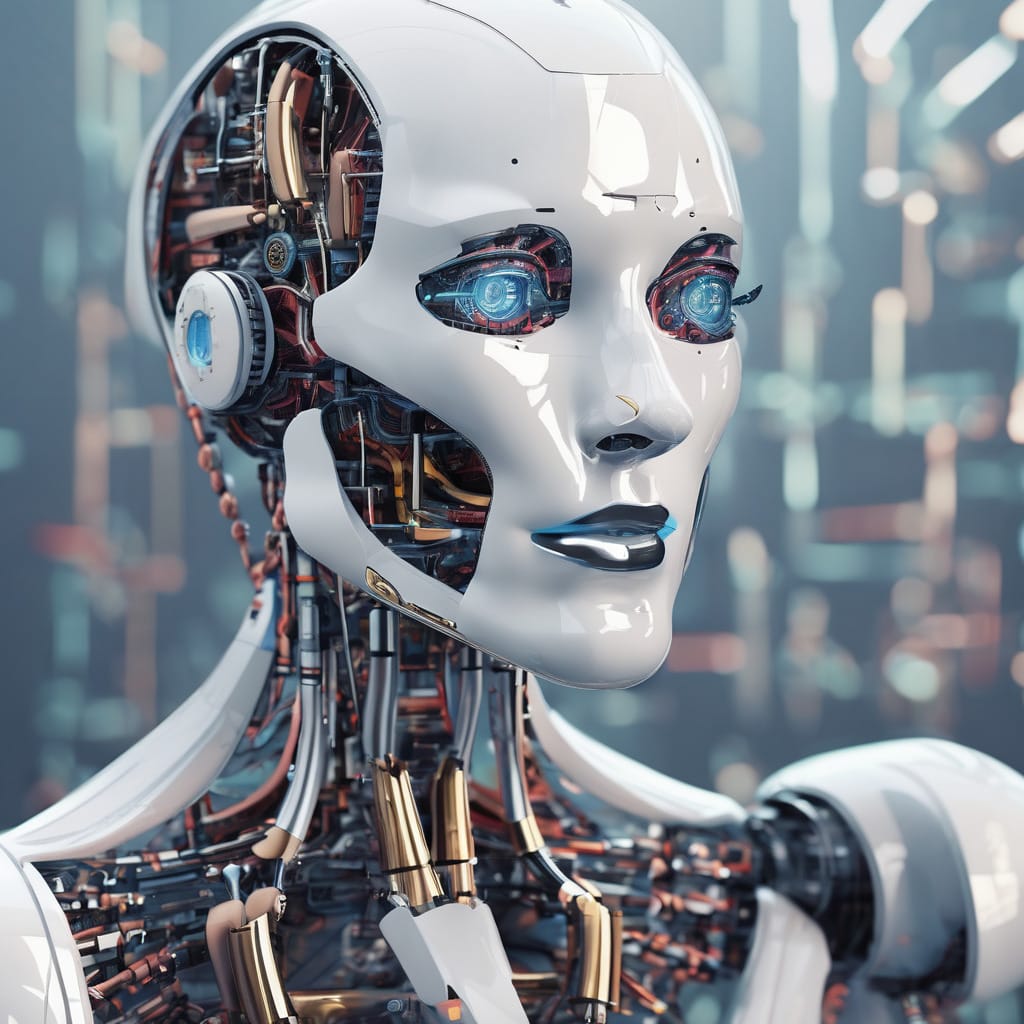
Large Language Models are a type of artificial neural network designed to process and generate human-like text. These models have revolutionized the field of natural language processing (NLP), enabling applications such as language translation, text summarization, and sentiment analysis.
Key Components of Large Language Models
- Encoder: The encoder takes in a sequence of words and converts them into a numerical representation.
- Decoder: The decoder generates a probability distribution over possible words, based on the input from the encoder.
- Attention Mechanism: The attention mechanism allows the model to focus on different parts of the input sequence, enabling it to capture long-range relationships.
- Large-Scale Training: Large Language Models are trained on massive amounts of text data, often in the order of millions or even billions of words.
How Large Language Models Work
- Masked Language Modeling: Large Language Models are trained using a technique called masked language modeling, where the model is trained to predict a missing word in a sentence.
- Sequential Data: Large Language Models process sequential data one word at a time, capturing temporal relationships.
- Hidden State: The hidden state stores information from the input sequence, enabling the model to capture complex patterns.
- Activation Functions: Large Language Models use activation functions such as softmax and ReLU to introduce non-linearity into the network.
Types of Large Language Models
- Transformer-based models: These models use a self-attention mechanism to process input sequences in parallel, making them highly efficient.
- Recurrent neural network (RNN) models: These models process input sequences sequentially, allowing them to capture temporal relationships.
- Hybrid models: These models combine the strengths of transformer-based and RNN-based models.
Advantages of Large Language Models
- Ability to Handle Long-Term Dependencies: Large Language Models can handle long-term dependencies in text data using both forward and backward passes.
- Ability to Handle Variable Length Inputs: Large Language Models can handle variable length inputs using both forward and backward passes.
- Good Performance on Simple Tasks: Large Language Models perform well on simple tasks such as language translation and text summarization.
Disadvantages of Large Language Models
- Training Complexity: Large Language Models can be difficult to train, especially for large datasets.
- Computational Complexity: Large Language Models can be computationally intensive, making them difficult to train and deploy.
- Overfitting: Large Language Models can suffer from overfitting, especially when using simple architectures.
Applications of Large Language Models
- Language Translation: Large Language Models are used for language translation tasks, such as machine translation and language translation systems.
- Text Summarization: Large Language Models are used for text summarization tasks, such as summarizing long pieces of text.
- Sentiment Analysis: Large Language Models are used for sentiment analysis tasks, such as analyzing the emotional tone of text.
Future of Large Language Models
- Increased Use in Real-World Applications: Large Language Models are expected to be used increasingly in real-world applications, such as language translation and text summarization.
- Improvements in Training Algorithms: Researchers are working on improving training algorithms for Large Language Models, such as using techniques like attention and memory-augmented networks.
- Increased Use in Multimodal Applications: Large Language Models are expected to be used increasingly in multimodal applications, such as speech and image processing.
Comparison with Other NLP Models
- Simple RNN: Large Language Models are more powerful than Simple RNNs, but require more computation.
- LSTM: Large Language Models are similar to LSTMs, but require more computation.
- GRU: Large Language Models are similar to GRUs, but require more computation.
I hope this helps! Let me know if you have any questions or need further clarification.