Neural Networks
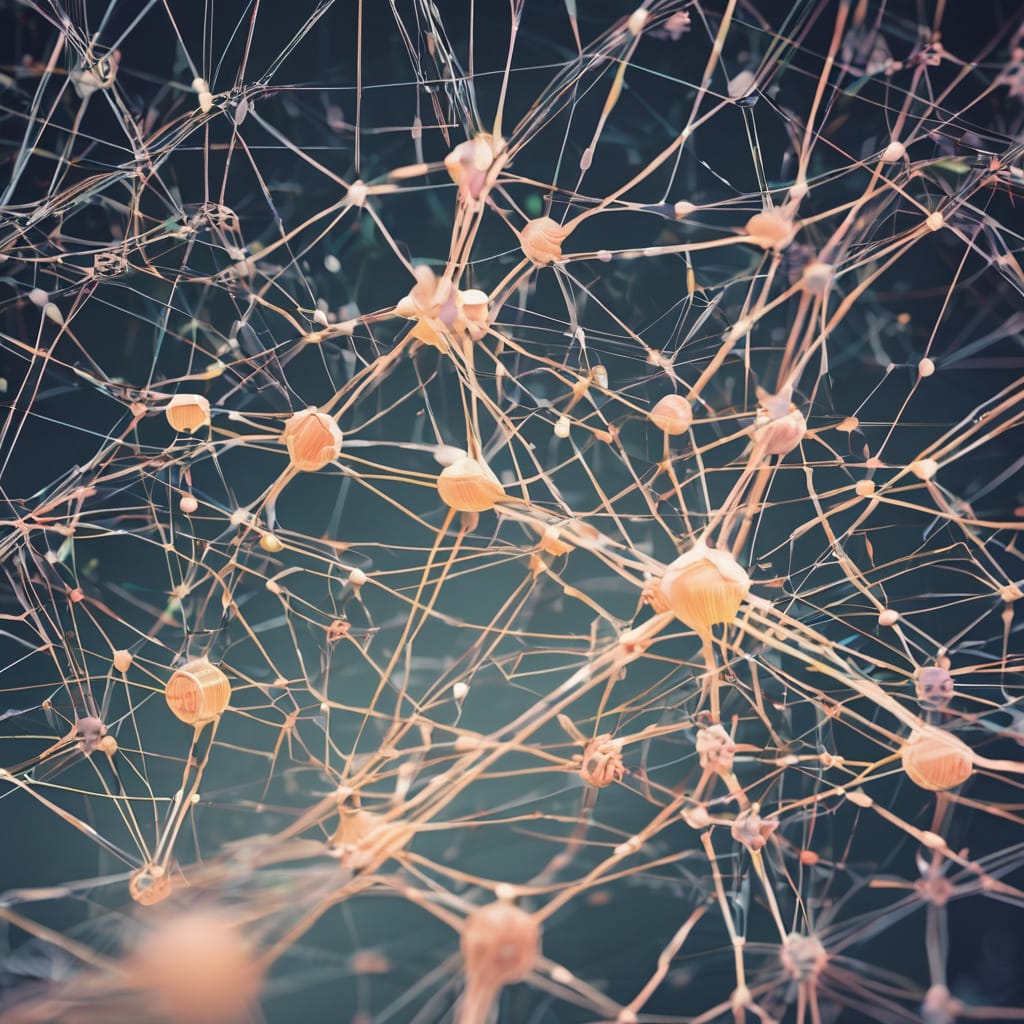
Neural Networks are a type of machine learning algorithm that uses a network of interconnected nodes or "neurons" to process and analyze data. They are a popular algorithm for classification, regression, and clustering tasks, and are known for their ability to handle complex and non-linear relationships.
What is a Neural Network?
A Neural Network is a type of machine learning algorithm that uses a network of interconnected nodes or "neurons" to process and analyze data. Each node applies a non-linear transformation to the input data, allowing the network to learn complex and non-linear relationships.
How Does a Neural Network Work?
A Neural Network works by using a network of interconnected nodes or "neurons" to process and analyze data. Each node applies a non-linear transformation to the input data, allowing the network to learn complex and non-linear relationships. The network is trained using a process called backpropagation, where the error between the predicted output and the actual output is used to adjust the weights and biases of the nodes.
Key Components of a Neural Network
- Nodes: The nodes are the basic building blocks of the network.
- Connections: The connections between the nodes allow the network to process and analyze data.
- Activation Functions: The activation functions are used to introduce non-linearity into the network.
- Loss Function: The loss function is used to measure the error between the predicted output and the actual output.
Types of Neural Networks
- Feedforward Networks: Feedforward networks are the most common type of neural network, where the data flows only in one direction.
- Recurrent Networks: Recurrent networks are used for sequence-based data, such as speech recognition and natural language processing.
- Convolutional Networks: Convolutional networks are used for image and video data.
Applications of Neural Networks
- Image Classification: Neural Networks are widely used for image classification tasks.
- Speech Recognition: Neural Networks are used for speech recognition tasks.
- Natural Language Processing: Neural Networks are used for natural language processing tasks.
- Robotics: Neural Networks are used in robotics to control and navigate robots.
Advantages of Neural Networks
- Ability to Learn Complex Relationships: Neural Networks can learn complex and non-linear relationships.
- Ability to Handle High-Dimensional Data: Neural Networks can handle high-dimensional data.
- Ability to Improve with More Data: Neural Networks can improve with more data.
Disadvantages of Neural Networks
- Requires Large Amounts of Data: Neural Networks require large amounts of data to train.
- Requires Significant Computational Resources: Neural Networks require significant computational resources to train.
- Can be Difficult to Interpret: Neural Networks can be difficult to interpret.
Common Applications of Neural Networks
- Deep Learning: Neural Networks are used for deep learning tasks, such as image and speech recognition.
- Natural Language Processing: Neural Networks are used for natural language processing tasks.
- Robotics: Neural Networks are used in robotics to control and navigate robots.
I hope this overview helps you understand Neural Networks better!