Predictive Maintenance
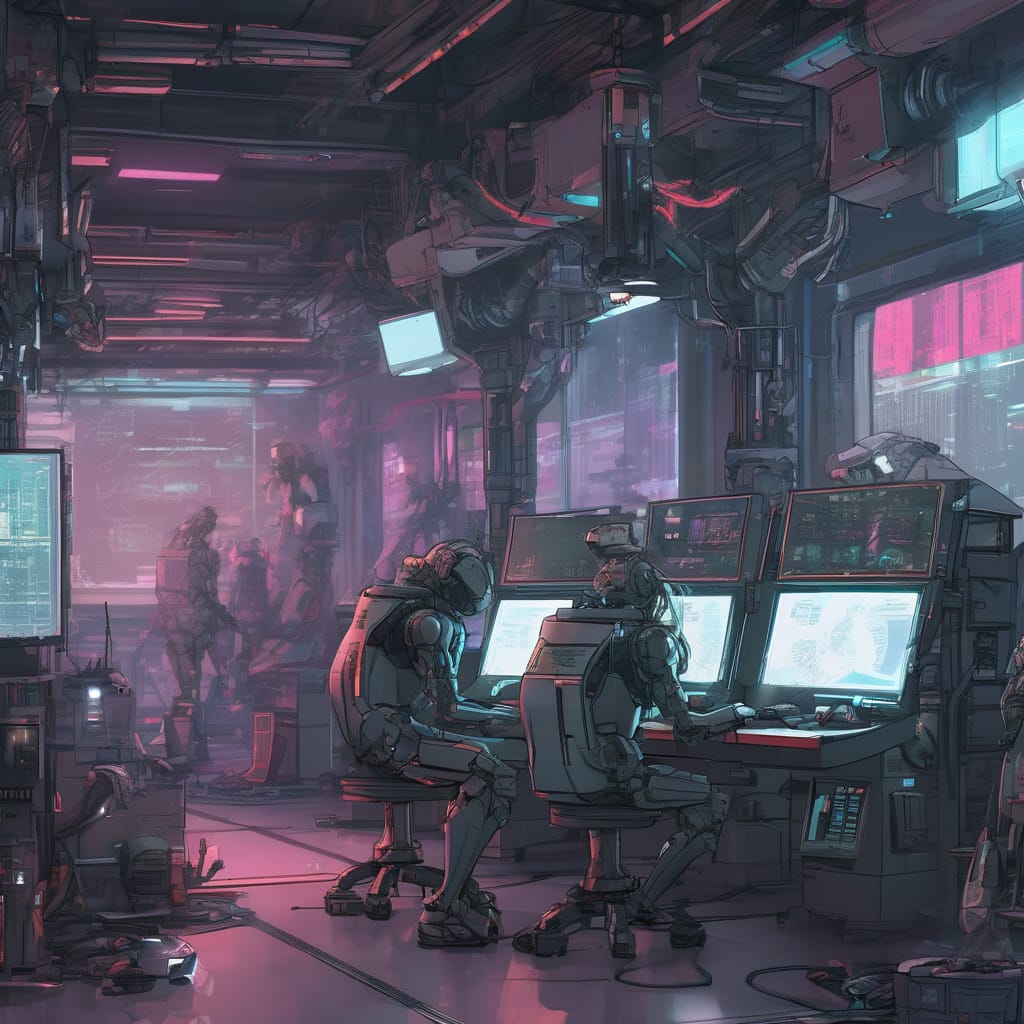
Predictive Maintenance is a type of maintenance strategy that uses data analysis and machine learning to predict when equipment is likely to fail or require maintenance. This approach is also known as Condition-Based Maintenance (CBM).
Key Components of Predictive Maintenance
- Sensor Data: Sensor data is used to monitor the condition of equipment and predict when maintenance is required.
- Machine Learning: Machine learning is used to analyze sensor data and predict when equipment is likely to fail or require maintenance.
- Data Analysis: Data analysis is used to identify patterns and trends in sensor data.
- Predictive Modeling: Predictive modeling is used to build models that predict when equipment is likely to fail or require maintenance.
Predictive Maintenance Tasks
- Equipment Monitoring: Equipment monitoring is the process of monitoring equipment condition using sensors and other data sources.
- Predictive Modeling: Predictive modeling is the process of building models that predict when equipment is likely to fail or require maintenance.
- Maintenance Scheduling: Maintenance scheduling is the process of scheduling maintenance based on predictive models.
- Root Cause Analysis: Root cause analysis is the process of identifying the root cause of equipment failures.
Applications of Predictive Maintenance
- Industrial Equipment: Predictive maintenance is used in industrial settings to predict when equipment is likely to fail or require maintenance.
- Transportation: Predictive maintenance is used in the transportation industry to predict when vehicles are likely to require maintenance.
- Manufacturing: Predictive maintenance is used in manufacturing to predict when equipment is likely to fail or require maintenance.
- Energy: Predictive maintenance is used in the energy industry to predict when equipment is likely to fail or require maintenance.
Benefits of Predictive Maintenance
- Reduced Downtime: Predictive maintenance can reduce downtime by predicting when equipment is likely to fail or require maintenance.
- Increased Efficiency: Predictive maintenance can increase efficiency by optimizing maintenance schedules.
- Cost Savings: Predictive maintenance can save costs by reducing the need for costly repairs and replacements.
- Improved Safety: Predictive maintenance can improve safety by predicting when equipment is likely to fail or require maintenance.
Challenges of Predictive Maintenance
- Data Quality: Data quality is a challenge in predictive maintenance, as high-quality data is required to build accurate models.
- Model Accuracy: Model accuracy is a challenge in predictive maintenance, as models must be highly accurate to be effective.
- Sensor Calibration: Sensor calibration is a challenge in predictive maintenance, as sensors must be accurately calibrated to provide accurate data.
Techniques Used in Predictive Maintenance
- Machine Learning: Machine learning is a technique used in predictive maintenance to build models that predict when equipment is likely to fail or require maintenance.
- Deep Learning: Deep learning is a technique used in predictive maintenance to build models that predict when equipment is likely to fail or require maintenance.
- Regression Analysis: Regression analysis is a technique used in predictive maintenance to build models that predict when equipment is likely to fail or require maintenance.
Evaluation Metrics for Predictive Maintenance
- Accuracy: Accuracy is a metric used to evaluate the accuracy of predictive models.
- Precision: Precision is a metric used to evaluate the accuracy of predictive models.
- Recall: Recall is a metric used to evaluate the accuracy of predictive models.
- F1-score: F1-score is a metric used to evaluate the accuracy of predictive models.
I hope this provides a high-level overview of Predictive Maintenance!