Recurrent Neural Networks (RNNs)
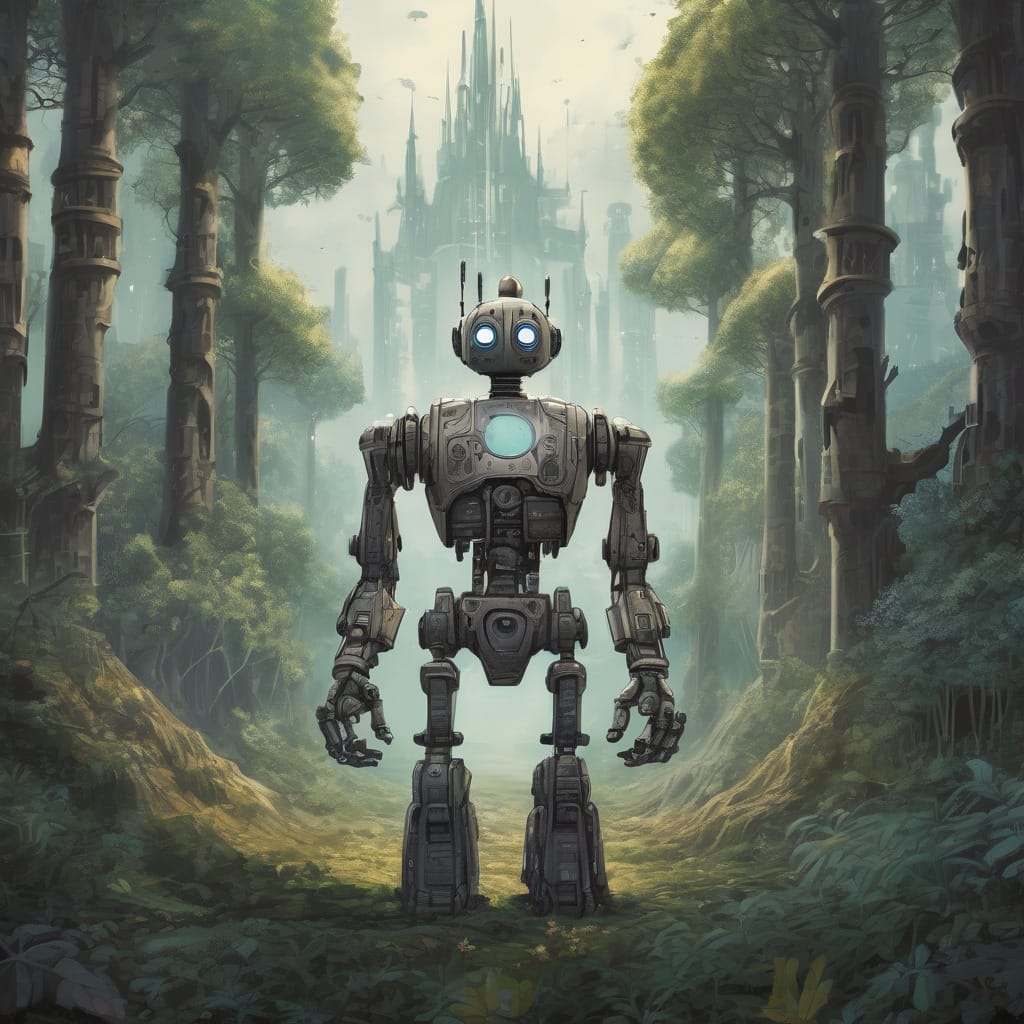
Recurrent Neural Networks (RNNs) are a type of Artificial Neural Network that uses recurrent connections to process sequential data.
Key Components
- Recurrent Connections: Connections that allow the output of a node to be fed back into the input of the same node.
- Hidden State: A hidden state that stores information from previous time steps.
- Activation Functions: Mathematical functions that determine the output of each node.
- Output Gate: A gate that controls the output of Recurrent Neural Networks.
Recurrent Neural Networks (RNNs) Architecture
RNNs typically consist of several layers of recurrent connections, with each layer processing the input sequence in a different way.
- Simple Recurrent Neural Networks: Use a single recurrent connection to process the input sequence.
- LSTM (Long Short-Term Memory) Networks: Use a special type of recurrent connection to process the input sequence, with a memory component that can store information for long periods of time.
- GRU (Gated Recurrent Unit) Networks: Use a special type of recurrent connection to process the input sequence, with a gate that controls the flow of information.
Training
RNNs are trained using a variety of algorithms, including:
- Backpropagation: An algorithm that adjusts the weights and biases of the nodes based on the error between the predicted output and the actual output.
- Stochastic Gradient Descent: An algorithm that adjusts the weights and biases of the nodes based on the error between the predicted output and the actual output, using a random subset of the training data.
- Regularization Techniques: Techniques that add penalties to the loss function to prevent overfitting.
Applications
Recurrent Neural Networks are widely used in many applications, including:
- Natural Language Processing: RNNs are used to process and understand human language, including text and speech recognition.
- Speech Recognition: Recurrent Neural Networks are used to recognize and transcribe human speech.
- Time Series Analysis: RNNs are used to analyze and predict time series data.
Advantages
- Ability to Learn Complex Patterns: Recurrent Neural Networks can learn complex patterns and relationships in sequential data.
- Ability to Handle Non-Linear Data: RNNs can handle non-linear data and relationships.
- Ability to Generalize: Recurrent Neural Networks can generalize well to new, unseen data.
Disadvantages
- Requires Large Amounts of Data: RNNs require large amounts of data to train and generalize well.
- Requires Significant Computational Resources: Recurrent Neural Networks require significant computational resources to train and evaluate.
- Difficult to Interpret: RNNs can be difficult to interpret and understand, making it challenging to identify the underlying patterns and relationships.